Unifying Paradigms (WP4)
Partners
KU Leuven, LIU, CNR, UCC, UNIROMA1, IST, UNIBO, CNRS, JSI, TUDa, UNIVBRIS, UNITN, VUB, CUNI, UArtois, CVUT, TU Delft, DFKI, EPFL, Fraunhofer, TU GRAZ, LU, PUT, RWTH AACHEN, CINI, UNIPI, VW AG, Imperial
See partner page for details on participating organisations.
People
Luc de Raedt (KU Leuven), WP leader.
About
The question to be answered in this research theme is How to integrate learning, reasoning and optimisation? The AI community has studied the abilities to learn, to reason and to optimize largely independent of one another, and has been divided into different schools of thought or paradigms. This is often described using confrontational terms such as: System 1 versus System 2, subsymbolic vs symbolic, learning vs reasoning, knowledge-based versus data-driven, model-based versus model-free, logic or symbolic versus neural, low vs high-level, etc.. No matter which terminology is used, the terms often refer to very similar distinctions and the state-of-the-art is that each paradigm can be used to solve certain tasks but not for others. For instance, the symbolic AI or the logic paradigm has concentrated on developing sophisticated and accountable reasoning methods, the subsymbolic or neural approaches to AI have concentrated on developing powerful architectures for learning and perception, and constraint and mathematical programming have been used for combinatorial optimisation. While deep learning provides solutions to many low-level perception tasks, it cannot really be used for complex reasoning; while for logical and symbolic methods, it is just the other way around. Symbolic AI may be more explainable, interpretable and verifiable, but it is less flexible and adaptable.
Trustworthy AI cannot rely on a single paradigm, it must have all the above mentioned abilities: it must be able to learn, to reason and to optimise. Therefore the quest for integrated learning, reasoning and optimisation abilities boils down to computationally and mathematically integrating different AI paradigms.
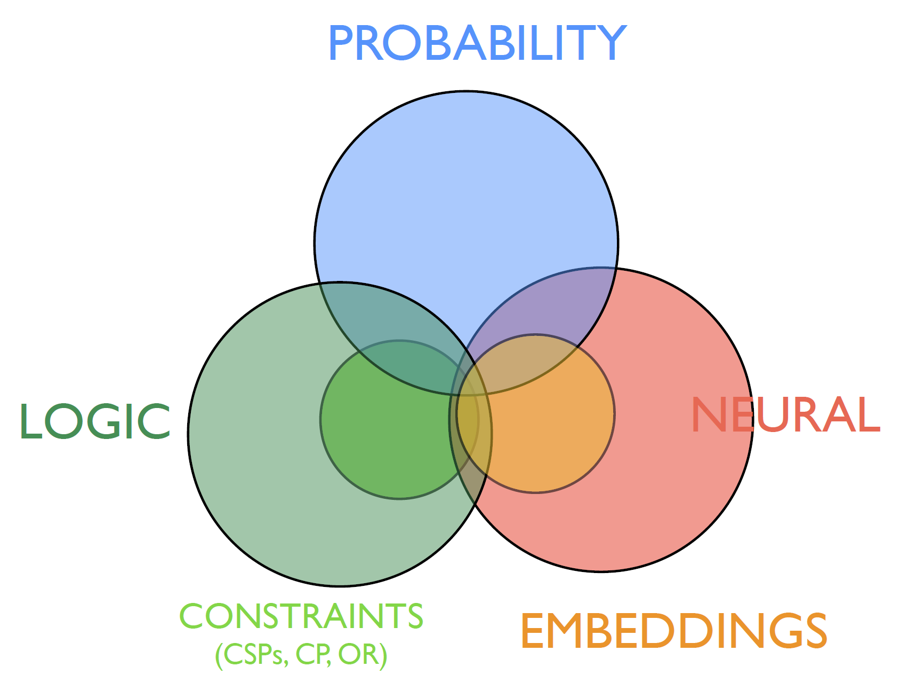
- Integrating or unifying different representations, i.e. (subsets of) representations — logic, probability, constraints, neural models, for learning and reasoning. Scaling up inference and learning algorithms for such representations. Example: develop NeSy system that can perform both logical inference and deep learning in the same way that pure logic and pure deep learning do.
- Explainability & Trustworthiness of integrated representations. Provide explanation methods that focus on understanding the rationales, contexts and interpretations of the model using domain knowledge rather than relying on the transparency of the internal computational mechanism.
- Learning for combinatorial optimisation and decision making. Example: learn and continuously adapt a model to schedule tasks in a data centre in order to minimize electricity consumption.
- Showcase applications in using domain knowledge in learning. Combining learning and complex reasoning (over knowledge graphs and ontologies). Example: improve the work on predicting sentence entailment where neural approaches have recently been debunked.
- Showcase applications in perception, spatial reasoning, robotics and vision. Combining high-level reasoning and low-level perception. Example: use common-sense knowledge to decide which item in an image is a real object and which is itself a picture.
Contact: Luc De Raedt (luc.deraedt@cs.kuleuven.be)
News related to WP4
-
IJCAI2023 Distinguished Paper Award to TAILOR Scientists from KU Leuven
Congratulations to Wen Chi Yang, Gavin Rens, Giuseppe Marra and Luc De Raedt on winning the IJCAI 2023 Distinguished Paper Award! The IJCAI Distinguished Paper Awards recognise some of the best papers presented at the conference each year. The winners were selected from among more than 4500 papers by the associate programme committee chairs, the programme and general chairs, and the president of EurAI.…
-
WP4 workshop at NeSy2023 conference in Siena, 3-5 July 2023
NeSy2023 conference (https://sites.google.com/view/nesy2023/home?authuser=0) will host a TAILOR WP4 workshop on July 3rd, 11:00-13:00, about “Benchmarks for Neural-Symbolic AI”. The workshop will be in hybrid format, however in-person participation is strongly suggested, especially for NeSy researchers and PhD students. For more information, we recommend to visit the webpage https://sailab.diism.unisi.it/tailor-wp4-workshop-at-nesy/. Abstract The study of Neural-Symbolic (NeSy) approaches has…
-
Explainability & Time Series Coordinated Action
TAILOR WP3 and WP4 would like to announce a new Coordinated Action focussed on Explainability & Time Series. The objective is to design novel methods for time series explanations and apply them into real case studies, such as high tide in Venice or similar. A first call for this Coordinated Action will be organised in…
-
Impressions from The Joint TAILOR-EurAI Summer school in Barcelona
During the week from 13th to 17th of June, the 19th EurAI Advanced Course on AI (ACAI) and 2nd TAILOR summer school was organised in Barcelona. This joint initiative was devoted to the themes of explainable and trustworthy AI and organized by Carles Sierra and Karina Gibert from the Intelligent Data Science and Artificial Intelligence Research Center at Universitat…
-
Accepted papers by TAILOR scientists to IJCAI-ECAI 2022
A lot of TAILOR scientists submitted their paperworks to the 31st International Joint Conference on Artificial Intelligence and the 23rd European Conference on Artificial Intelligence (IJCAI-ECAI 2022). The conference will be in Vienna, from 23rd to 29th of July, 2022. Here below the (not full) list of accepted papers presented by people involved in TAILOR: AISafety…
-
AAAI 2022: accepted papers from the TAILOR Network
The AAAI is one of the major global conferences for AI research and scientific exchange among AI researchers, practitioners, scientists, and engineers in affiliated disciplines. In 2022, it will be a virtual conference February 22 – March 1. Several prominent researchers from the TAILOR consortium have papers accepted for the conference: The (very long) list…