Trustworthy AI – WP3
Partners
CNR, LIU, INRIA, UCC, UNIROMA1, IST, UNIBO, TU/e, CNRS, UNIVBRIS, UNITN, CEA, UArtois, TU Delft, DFKI, EPFL, LU, PUT, CINI, slovak.AI, UNIPI, UGA, UPV, VW AG, ENG
See partner page for details on participating organisations.
People
Umberto Straccia (ISTI-CNR, WP leader), Fosca Giannotti (ISTI-CNR), Francesca Pratesi (ISTI-CNR)
About WP3
Explainability, Safety, Fairness, Accountability, Privacy, and Sustainability are the dimensions of Trustworthy AI that are necessarily intertwined with the foundation themes of the project through a continuous mutual exchange of requirements and challenges to develop legal protection and value-sensitive approaches. The questions that will drive the research are:
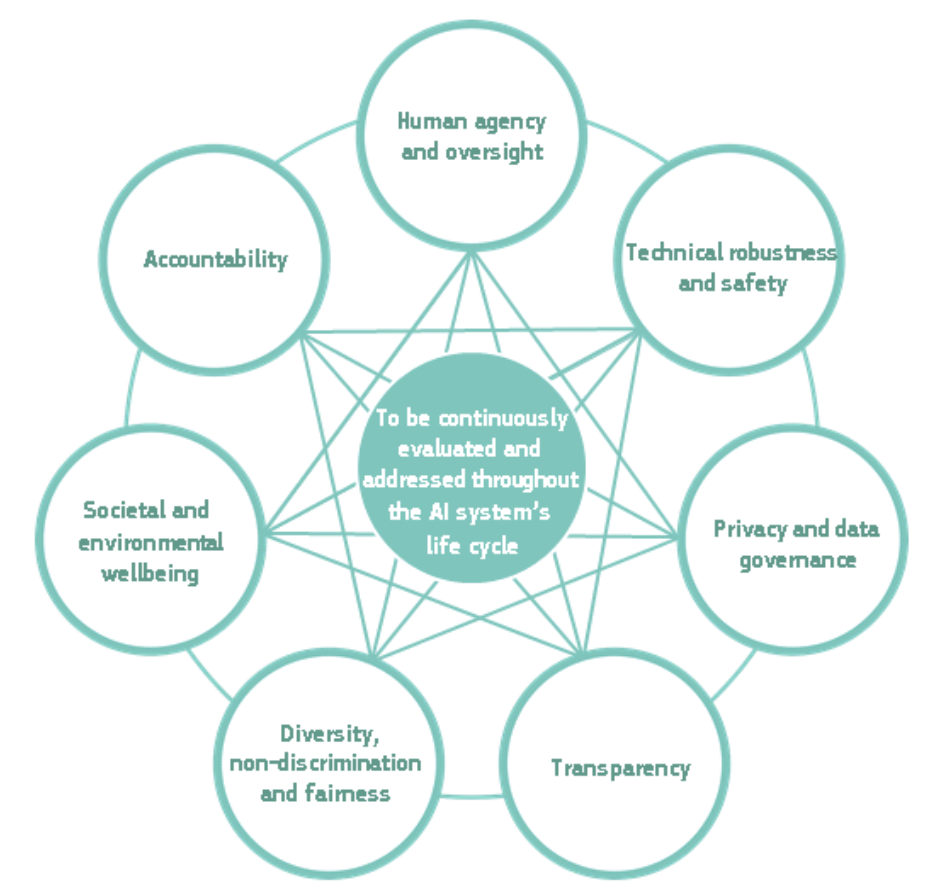
- How can we guarantee user trust in AI systems through explanation? How to formulate explanations as Machine-Human conversation depending on context and user expertise?
- How to bridge the gap from safety engineering, formal methods, verification as well as validation to the way AI systems are built, used, and reinforced?
- How can we build algorithms that respect fairness constraints by design through understanding causal influences among variables for dealing with bias-related issues?
- How to uncover accountability gaps w.r.t. the attribution of AI-related harming of humans?
- Can we guarantee privacy while preserving the desired utility functions?
- Is there any chance to reduce energy consumption for a more sustainable AI and how can AI contribute to solving some of the big sustainability challenges that face humanity today (e.g. climate change)?
- How to deal with properties and tensions of the interaction among multiple dimensions? For instance, accuracy vs. fairness, privacy vs. transparency, convenience vs. dignity, personalization vs. solidarity, efficiency vs. safety and sustainability.
Coordinated Actions and tasks
Coordination Actions (CA) are groups of researchers convened around a specific topic of interest, to start, to investigate, to promote and to accelerate Trustworthy AI. Interested to join? Please take a look at the current CA proposals. You may join to some existing one, just contact the CA leader, or propose one by yourself. To do so, contact the WP3 Task Leader of the task that is predominant in your proposal.
T3.1 Explainable AI Systems
Comparison of methods for interpretation of convolutional neural networks for the classification of multiparametric MRI images on unbalanced datasets. Case study: prostate cancer, vestibular schwannoma cancer
Tasks: T3.1 Explainability , T3.7 Trustworthy AI as a whole, T4.3: Learning and reasoning with embeddings, knowledge graphs & ontologies
Partners: CNR, UNIPI, UGA, INRIA, LIRA
Explainable malware/security threat detection: Comparison of methods for detection and prediction of malware/security attacks that are able to produce some kind of explanation or characterization of the attack
Tasks: T3.1 Explainability, T3.7 Trustworthy AI as a whole, T4.3: Learning and reasoning with embeddings, knowledge graphs & ontologies
Partners: Slovak.AI, CNR
T3.2 Safety and Robustness
Dealing with truly adversarial examples
Tasks: T3.2 Safety and Robustness , T3.4 Accountability and Reproducibility
Partners: VRAIN/UPV, JRC-EC/VRAIN,, Slovak.AI, CNR
Robust Evaluation: prevent specialisation and test replacement
Tasks: T3.2 Safety and Robustness , T3.4 Accountability and Reproducibility
Partners: VRAIN/UPV, JRC-EC/VRAIN, CEA
SafeAI and AISafety workshops (AAAI and IJCAI)
Tasks: T3.2 Safety and Robustness , T3.8 Fostering the AI scientific community around Trustworthy AI
Partners: CEA, VRAIN/UPV, UNIPI, CNR, TUDelft
Formal methods and V&V for AI
Tasks: T3.1 Explainability, T3.2 Safety and Robustness , T3.4 Accountability and Reproducibility, T3.7 Trustworthy AI as a whole, T3.8 Fostering the AI scientific community around Trustworthy AI
Partners: CNR, VRAIN/UPV
T3.3 Fairness, Equity, and Justice by Design
Operationalizing Fairness Metrics
Tasks: T3.3 Fairness, Equity, and Justice by Design
Partners: UNIPI, INRIA, TUDelft
T3.4 Accountability and Reproducibility
Emergent responsibility in reproducible multi-agent settings
Tasks: T3.4 Accountability and Reproducibility, T6.4 Emergent Behaviour, agent societies and social networks
Partners: TUDelft
Holistic assessment and certifications of AI systems for reproducibility and accountability
Tasks: T3.2 Safety and Robustness , T3.4 Accountability and Reproducibility, T3.7 Trustworthy AI as a whole
Partners: CNR, TUDelft, PUT, TUE
T3.5 Respect for Privacy
Impact on Fairness of Privacy Preserving Data Transformation
Tasks: T3.3 Fairness, Equity, and Justice by Design, T3.5 Respect for Privacy
Partners: UNIPI, UGA, LiU, Inria
Challenges for Guaranteeing Privacy while Preserving Utility
Tasks: T3.5 Respect for Privacy, T3.7 Trustworthy AI as a whole
Partners: DFKI, EPFL, INRIA, LIU
Automatic Tools for Analyzing and Explaining Privacy Risks
Tasks: T3.1 Explainability, T3.5 Respect for Privacy, T3.7 Trustworthy AI as a whole
Partners: UGA, CNR, UNIPI, INRIA
T3.6 Sustainability
Probabilistic Workload Forecasting in Cloud Computing using Deep Learning approaches
Task: T3.6 Sustainability
Partners: UCC
News related to WP3
-
Video Series: Trustworthy AI Explained
The TAILOR network has produced a series of videos, based on the major chapters of the TAILOR Handbook of Trustworthy AI. The videos are available on YouTube for anyone seeking independent, qualified and accessible information about Trustworthy AI.
-
Explainability & Time Series Coordinated Action
TAILOR WP3 and WP4 would like to announce a new Coordinated Action focussed on Explainability & Time Series. The objective is to design novel methods for time series explanations and apply them into real case studies, such as high tide in Venice or similar. A first call for this Coordinated Action will be organised in…
-
TAILOR Handbook of Trustworthy AI
The TAILOR Handbook of Trustworthy AI is an encyclopedia of the major scientific and technical terms related to Trustworthy Artificial Intelligence. The main goal of the Handbook of Trustworthy AI is to provide non experts, especially researchers and students, an overview of the problem related to the development of ethical and trustworthy AI systems. The…