The TAILOR Handbook of Trustworthy AI is an encyclopedia of the major scientific and technical terms related to Trustworthy Artificial Intelligence.
The main goal of the Handbook of Trustworthy AI is to provide non experts, especially researchers and students, an overview of the problem related to the development of ethical and trustworthy AI systems.
The coordinators of this ambitious work are Umberto Straccia and Francesca Pratesi, from Institute of Information Science and Technologies “A. Faedo” of the National Research Council of Italy (ISTI-CNR). When we asked to Francesca to give an overview of their work, she stated:
Trustworthy AI is a term that comprehends a variety of different dimensions, namely explainability, safety, fairness, accountability, privacy, and sustainability. Some of them (such as security and privacy protection) are more consolidated, while other ones (e.g., explainability and sustainability) are relatively newer. Nevertheless, we acknowledge a certain lack of a common ground in both terms and definitions. Indeed, our ambition is to create a common language, starting from existing taxonomies and definitions whether is possible, and going further in the hierarchies of various concepts. This handbook will be beneficial to both beginners (who can learn from the basis of the topic, and will particularly take advantage from summaries and examples) and specialists (who can go more in depth with also suggested readings, links, and bibliographies, and who can ask to contribute in the project given the living nature of the handbook).
To facilitate its consultation, authors choose an encyclopedia-like structure and is presented in the form of a publicly accessible WIKI. In particolar, in this Encyclopedia you can find definition related to:
- Explainable AI. Here you can find an overview of the main properties that an explanation should have and of the several methods to provide multimodal explanations;
- Safety and Robustness, in which the challenges of developing AI systems that are safe, reliable, and robust is faced, and a way to evaluate this aspects in practice is provided;
- Fairness, Equity, and Justice by Design. In this chapter, authors reflects upon the grounds of discrimination, and how a bias or segregation can be defined; then, they make a step in defining what fair machine learning could be, and what are the metrics we can adopt to measure (un)fairness.
- Accountability and Reproducibility. Here, they analyze the two souls of this topic, i.e., the two interrelated concepts of Accountability and Reproducibility: the former term is more related to responsibility, blameworthiness, liability, and prevent misuse, where the latter term is more related to measures, quality standards, and procedures to model the development of learning methods for AI.
- Respect for Privacy. This section provides an overview of the main attack that can put at risk individual privacy, and they explain the difference between pseudonymization and actual anonymization, also describing the main family of privacy models.
- Sustainability. The last chapter of the Handbook is focused on one of the newest challenge that our society is facing, in particular, to provide solutions for optimizing both the resources used in AI systems and the computation itself.
Finally, in the last section you can find an Index, in which a list of all the entries in alphabetical order is provided alongside a short definition of each term, a map to find the term in the handbook, and a link to deepen the concept.
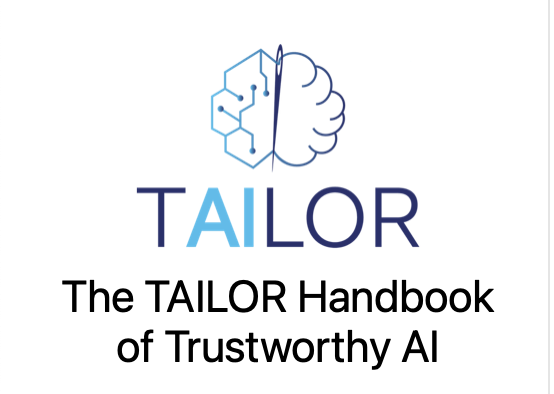
The Handbook can be found on TAILOR website at this link: http://tailor.isti.cnr.it/handbookTAI/TAILOR.html