On the 10th of May 2022, the fourth joint “Theme Development Workshop” (TDW) focused on the latest trends and challenges of “AI for Future Manufacturing”. The discussion hosted close to 80 key experts from 20 European countries, from both academia and the manufacturing sector, as well as other relevant stakeholders. The participants had one primary, but still very challenging goal – to identify common goals between academia and the manufacturing sector, and define promising approaches for European research and innovation in Trustworthy AI.
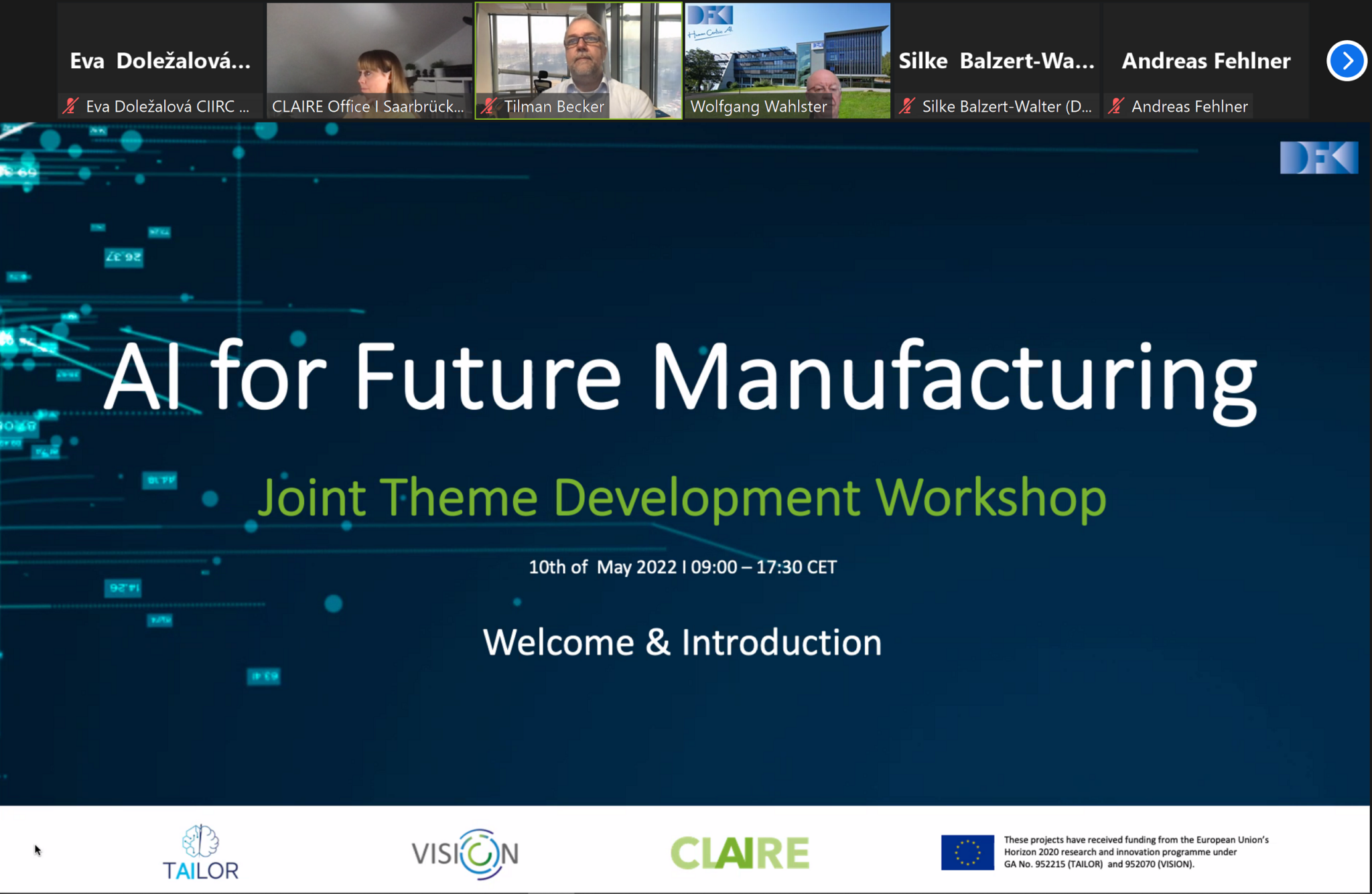
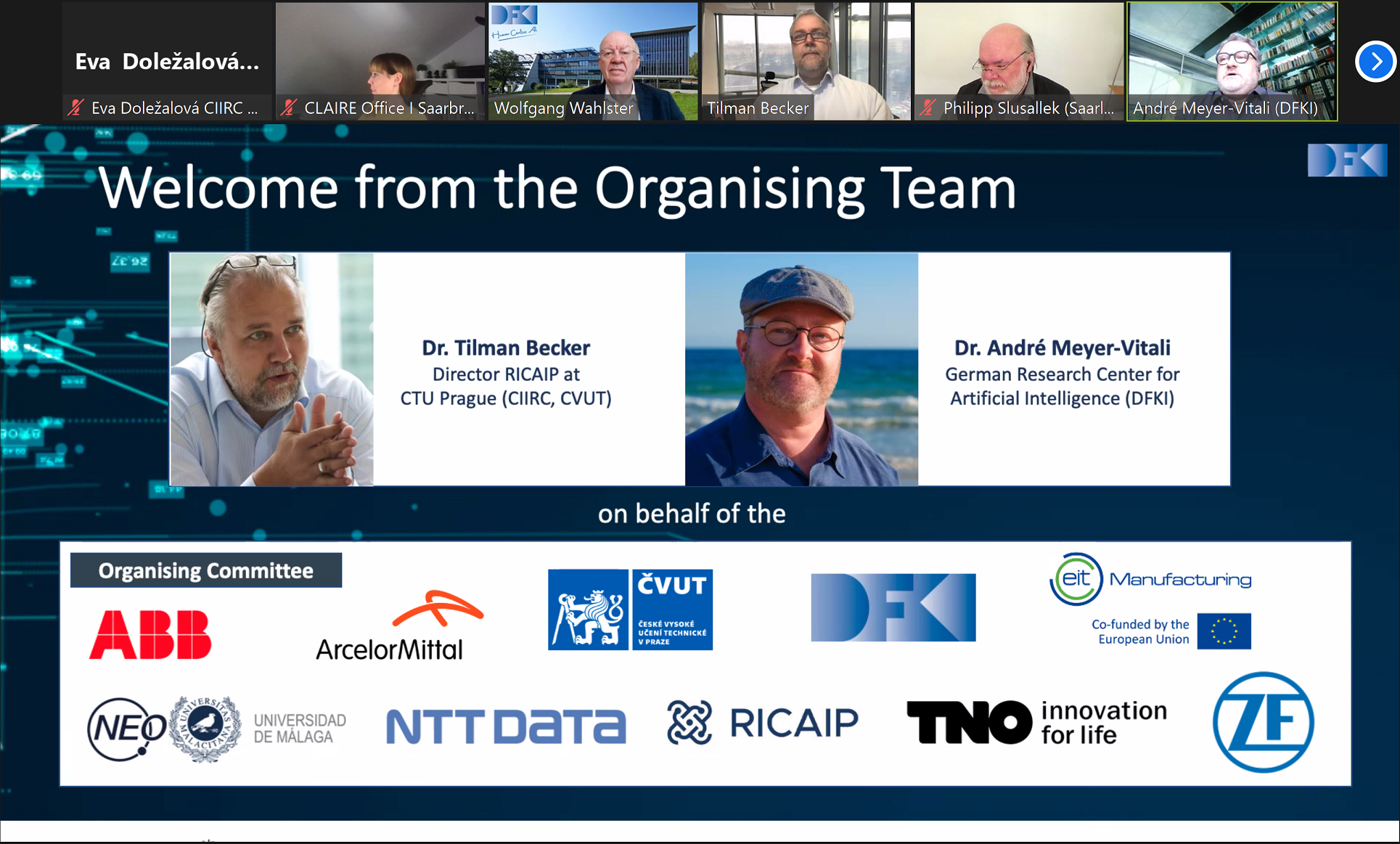
The discussion was opened by Prof. Wolfgang Wahlster, CEA of DFKI and the scientist among the three fathers of Industrie 4.0, who developed the concept in 2010 and co-authored the first paper about the fourth industrial revolution in 2011. Prof. Wahlster outlined the challenges that European industry and society had to face over the last decade. At the same time, these challenges, such as a lack of skilled workforce, an ageing society pushing on later retirement, or very volatile markets that need multiadaptive factories as the products change quickly within the short product lifecycles, have been socio-economic drivers of Industry 4.0 and industrial AI. There has been a need for increasing product variability leading to mass customisation with low-volume and high-mixture factories that shall be based on a resource-efficient, green, circular economy.
“Not only deep learning but also deep understanding will be significant for the next decade of Industrie 4.0 as we strive for long-term autonomy of machines in smart factories,” Prof. Wolfgang Wahlster pointed out and reminded the audience that trust in Industrie 4.0 methods could only be built up with factory operators during the last decade, because data-driven machine learning has been combined with symbolic models capturing physical constraints as well as context, task or process models. Deep learning is not enough for the next generation of Industrie 4.0 systems – hybrid AI systems are needed. AI technologies are a key success factor for Industrie 4.0 since machine learning disrupts sensor interpretation for predictive maintenance and online quality control.
Prof. Wahlster shared his vision of the use of AI in robotics that will enable the physical assistance of human workers by cobots, accompanied by the cognitive assistance by softbots as virtual characters. Modern technology shall empower the workers with assistive and smart tools and enhance their flexibility rather than replace them in the manufacturing process. Among other goals for the next step of Industrie 4.0, Prof. Wahlster also discussed zero-error production based on incremental real-time quality monitoring which is already at the top of the agenda of big production companies. Zero-error means that there are no incorrect actions by either workers or robots which remain undetected. The same applies for all variants of interaction between humans, human and robot or robot and robot. The system immediately detects errors by plan recognition, interaction recognition or intention recognition through the use of AI for real-time interpretation of relevant sensor data. The new concept is to use many small quality management loops in the production process so that in every production step, potential error effects can be corrected on the spot.
“Industrie 4.0 brings many AI subfields together in one of the most important fields of industrialised European countries,” Prof. Wahlster finished his inspiring talk.
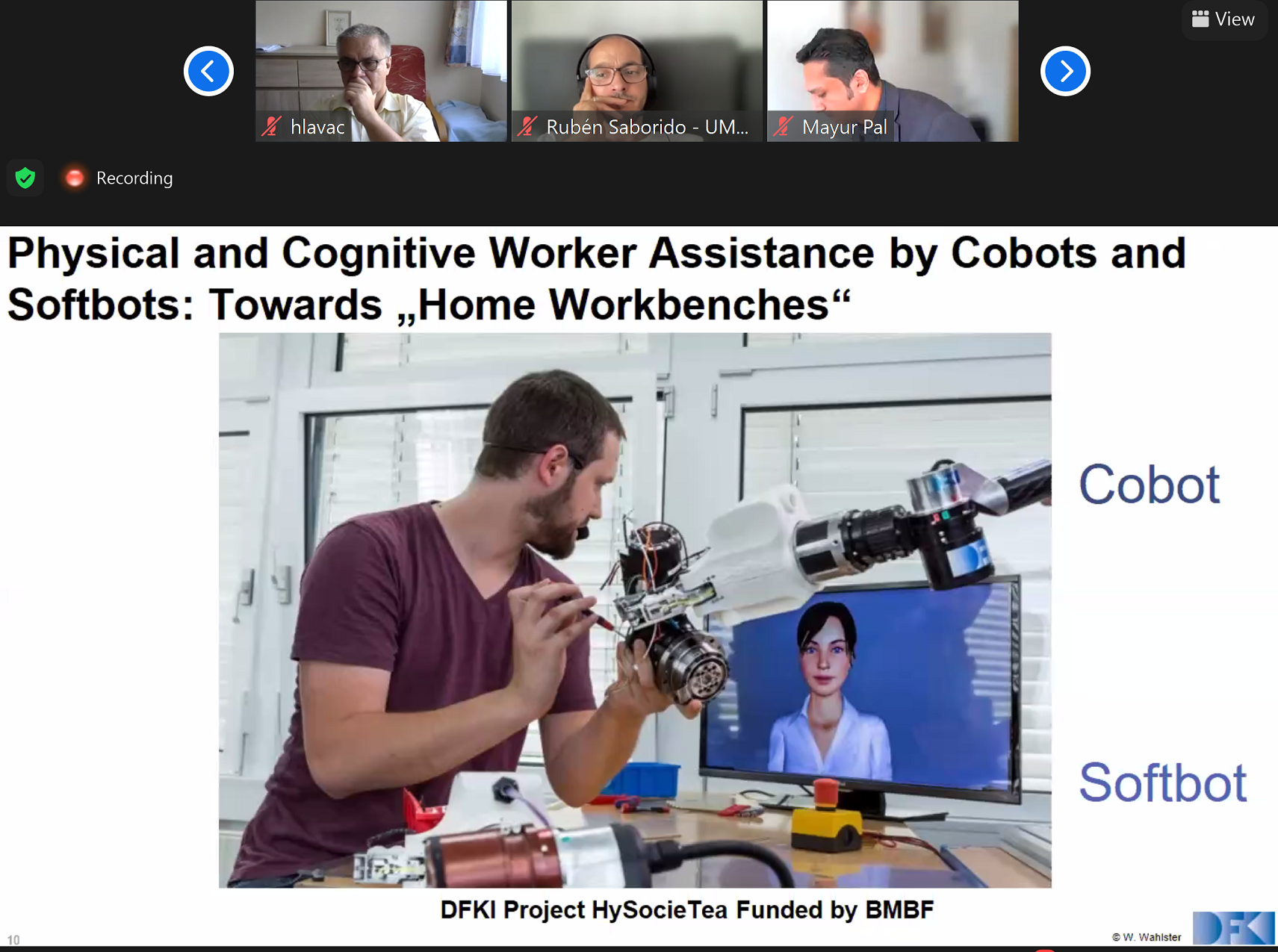
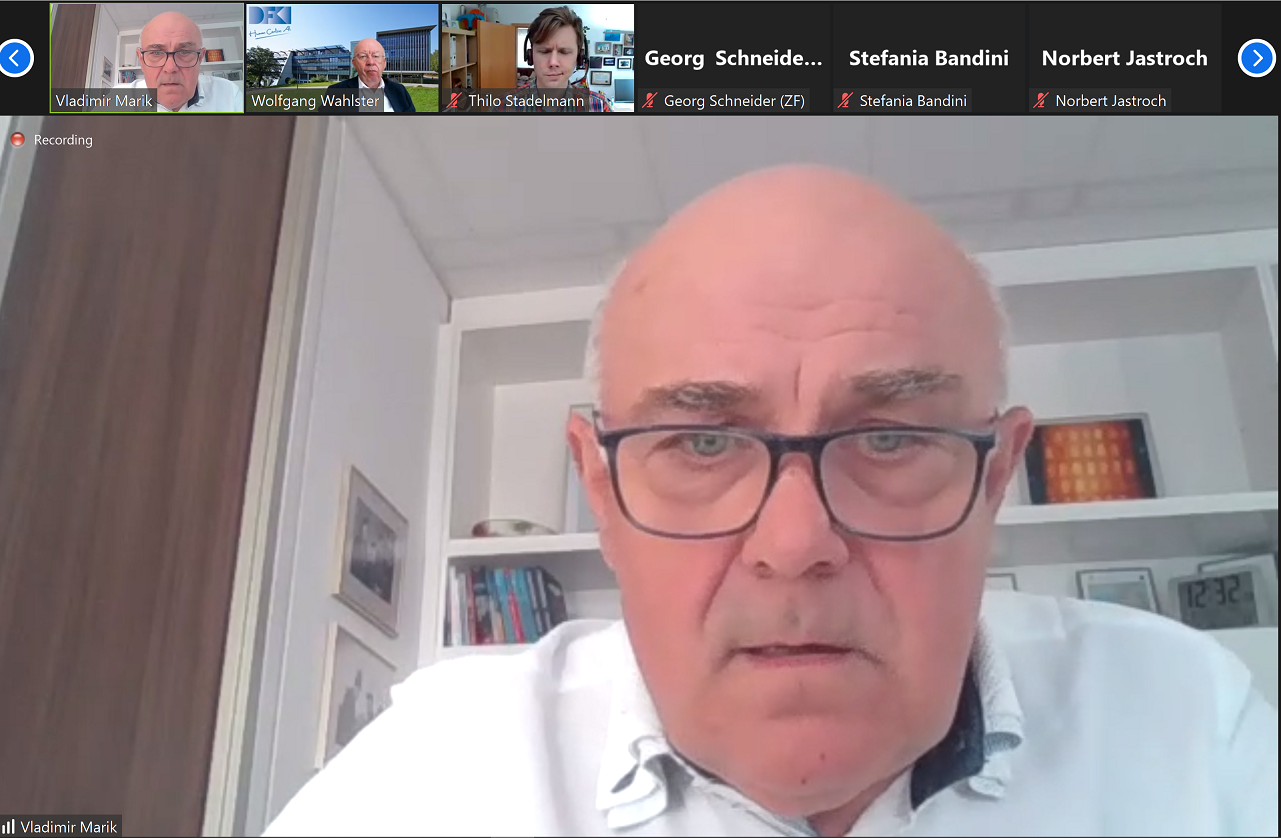
In the afternoon, a close collaborator of Prof. Wahlster, Prof. Vladimír Mařík, Scientific Director of the Czech Institute of Informatics, Robotics and Cybernetics Czech Technical University in Prague, spoke about building the AI ecosystem in the national and European context, and about specific aspects of Phase 2 of Industry 4.0. From a scientific point of view, the self-learning and adaptability of learning data are important for machine learning systems in the long term. The latest enhancements of the process-oriented digital twins allow reliable prediction and simulation of machine tool behaviour in the machining process. As the industry requires more complex solutions, we are in the phase of optimisation and machine learning towards SELF-X functionalities of AI: Self-learning, self-adaptability or self-development AI. Overall, the Industry 4.0 Phase 2 needs global flexible, self-learning engineering solutions enabling the understanding, controlling, and managing of very complex manufacturing systems and their environments. In some way, these AI-based systems should understand what they are doing and manage in a very intelligent way what their own development learnt from previous experience.
“Knowledge structures and models are expected to play the dominant role,” said Prof. Mařík, continuing: “It is also necessary to bridge the gap between the academic research environment and industrial needs by a specialised AI system integration industry using adequate computing and data storage facilities.”
New highly specialised AI companies can explore dedicated physical computational resources and data storage facilities. The use cases performed, industrial data collected, and AI algorithms developed have to be stored in shared data space and used in an innovative and creative way by these highly specialised AI companies to tailor new industrial solutions and build adequate computational and data resources. At the same time, standardisation and interoperability are an absolute must.
Therefore, all main stakeholders and professional initiatives shall work together on creating a joint physical coworking data space to achieve higher re-usability of data based on rules and standardisation. On the other hand, the current AI research ecosystems are not adequate enough for the know-how transfer and implementation to the industry. The computing and data-storage requirements for storing, reusing, and integrating complex AI solutions are increasing rapidly.
The 77 participants (41 academia, 33 industry, and 3 other) from 19 countries were divided into 14 breakout sessions according to various topics, including fundamental topics such as “Trustworthy AI in manufacturing” and “Federated Learning”, more cross-cutting topics such as “Human-robot and human-machine interaction in manufacturing processes”, “Optimisation” and “AI in product development”, and more specific topics such as “Retrofitting of AI” or “Smart manufacturing for space applications”. In smaller groups, the participants identified key elements, challenges, and recommendations on future strategic developments relevant to each topic. Despite the relatively large number of breakout sessions, there are still more untouched topics that deserve attention for future TDWs.
The workshop was co-chaired and moderated by Dr. André Meyer-Vitali, senior researcher at DFKI, and Dr. Tilman Becker, RICAIP director, CIIRC CTU in Prague.
The event was co-organised by VISION, the coordination and support action for the ICT-48 Networks, and the European AI initiative CLAIRE AISBL, under the lead of the network of research excellence centres TAILOR. The organising committee, composed of academic and industrial representatives from each partner, put together an interesting programme of inspiring keynotes followed by breakout sessions on current topics in manufacturing.