Machine learning and other AI technologies have the potential to greatly increase scientific output in many domains, while even increasing quality and lowering costs. However, due to the current exponential growth and influx of investment and interest, access to computer scientists and machine learning specialists is often a limiting factor. Scientists working in the group of Holger Hoos work on AutoAI, solutions that will democratise AI by allowing experts from other domains to develop their own AI tools.
Scientists from all domains working with large amounts of data face similar challenges. Curating, reading and interpreting data is time-consuming and tedious. Humans also tend to make errors and introduce biases.
Computational solutions to this problem were introduced in the early seventies. Typically, domain experts have developed task-specific models that can successfully handle a specific data analysis. However, these models are not easily transferable to other tasks or domains, and any change in the task or equipment often implies the re-design of the whole model
– For example, switching from one satellite to another might make your model unusable, forcing you to design a new one, explains Julia Wąsala. With AutoAI methods, you don’t have to redesign a whole new system each time.
In earth observation studies, domain experts use data from sophisticated instruments in space to tackle today’s greatest environmental challenges, such as climate change and air pollution. One such phenomenon is the release of greenhouse gases.
Typically, scientists interpret and organise satellite data to combine it with data from maps and meteorological information. With the help of machine learning, this process can be made faster, more accurate and less biased.
– The problem is that you need a lot of machine learning experience to be able to design machine learning models for these applications, says Wąsala. With AutoAI algorithms, earth observation scientists will have access to smart algorithms that design the models for them.
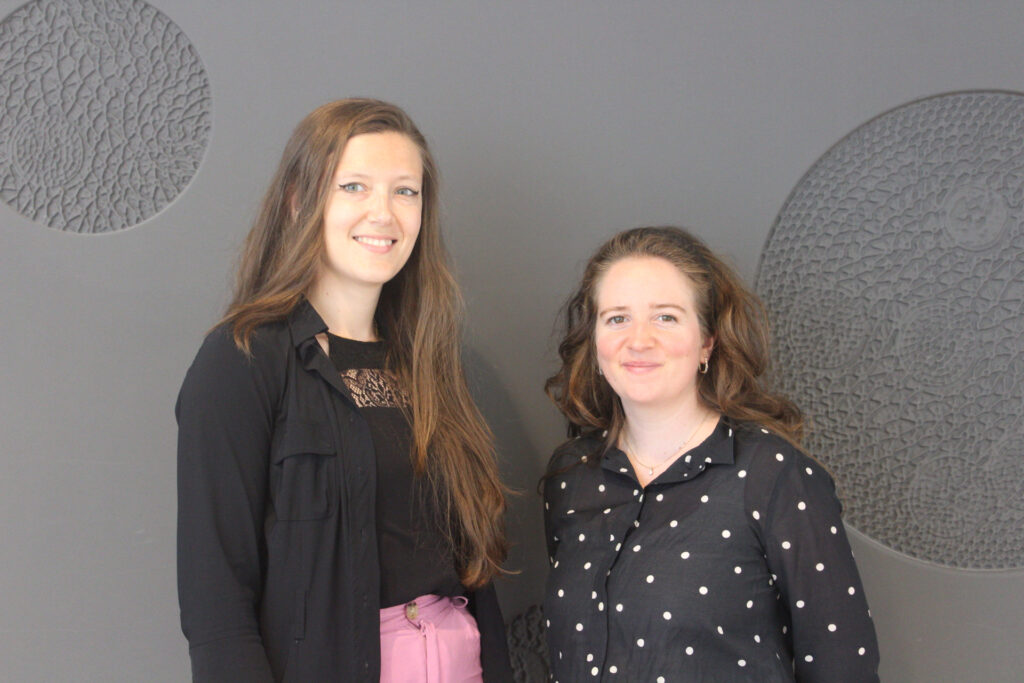
Julia Wąsala (left) and Annelot Bosman
The scientist provides the data and the AutoAI assembles modules, like building blocks, and then assembles the best possible model for the data. The AutoAI actually takes away a lot of choices you otherwise have to make and are tricky to make for non-experts.
Using AutoAI methods to create new models not only increases the possibilities for scientists outside the machine learning sector to build their own models, but it also has the potential to increase the trustworthiness of the models.
– A huge problem in machine learning, in particular neural networks, is that they can be easily fooled. To make sure that doesn’t happen, the field of verification has emerged. Verification is very expensive, so what we’re working on is making AutoAI do the verification to make sure the neural network is safe, explains Annelot Bosman.
Eventually the researchers would like to incorporate verification features in a framework that would create a neural network that is already safe from the beginning and ready for use without additional verification.
AutoAI technology also allows for using less computational power and energy. Usually, AI models need extensive training and testing but in AutoAI, smart algorithms do the testing. This reduces the time spent using supercomputers, freeing valuable computational power for other applications and reducing energy use.
– With AutoAI frameworks, users don’t need to have in-depth programming knowledge, but they need to understand machine learning in theory. And that can be learnt in class, concludes Bosman.
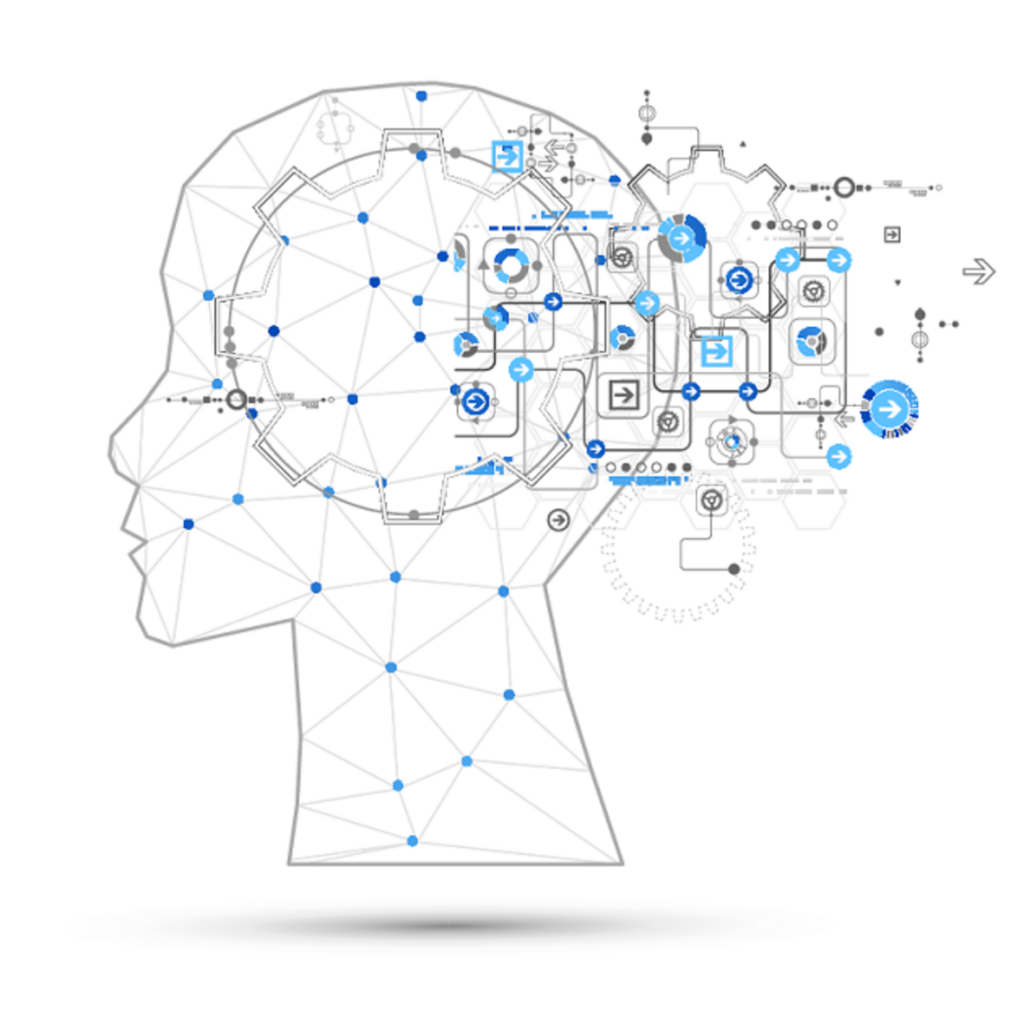
NEURAL NETWORKS
Artificial neural networks are models inspired by the biological neural network in the animal brain. It contains different nodes, connected by edges and assembled into layers. The term deep learning, or deep neural networks, refers to the use of multiple layers.
About the researchers:
Julia Wąsala, PhD student in Automated Machine Learning for Earth Observation, supervised by dr. Mitra Baratchi and Annelot Bosman, PhD student in Neural Network Verification, supervised by dr. Jan van Rijn. They all work with Holger Hoos at Leiden University, the Netherlands.
Holger Hoos, is an Alexander von Humboldt Professor of Artificial Intelligence, RTWH Aachen University, Germany, and Professor of Machine Learning, Leiden University, The Netherlands. Hoos is also an Adjunct Professor of Computer Science at the University of British Columbia, Canada and the Chair of the Board for the Confederation of Laboratories for AI Research in Europe (CLAIRE).
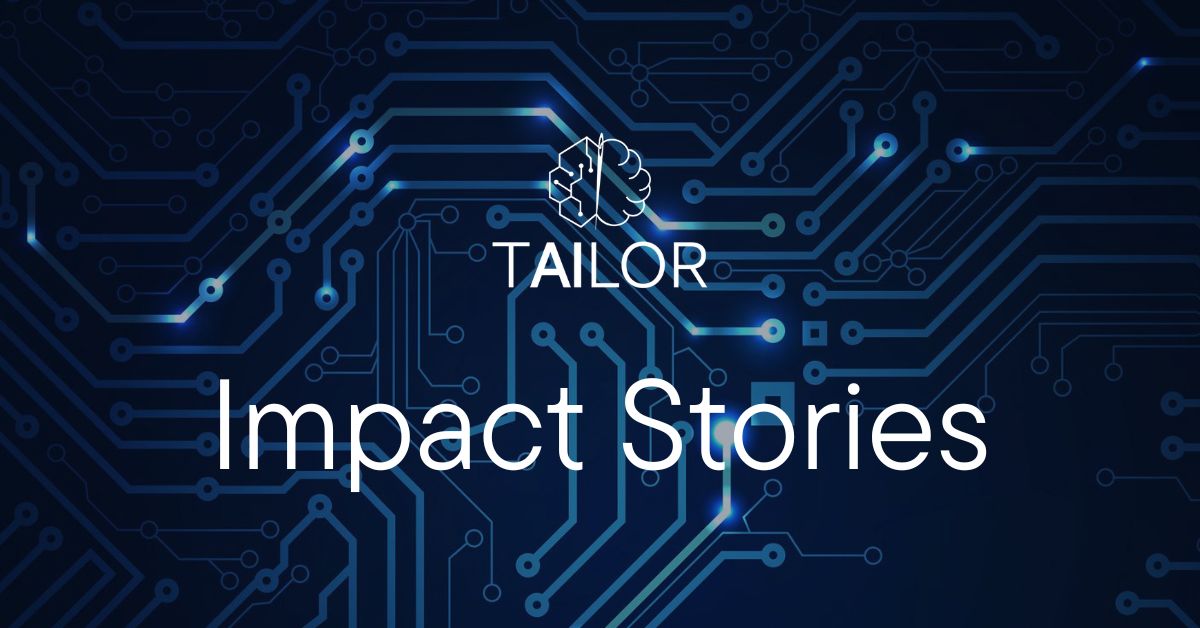