We are thrilled to announce that TAILOR is starting a new section!
Every month, we want to acknowledge some valuable TAILOR papers, selected among the papers published by scientists belonging to our network by TAILOR principal investigator Fredrik Heintz.
The list of the most valuable papers gathers contributions from different TAILOR partners, each providing valuable insights on different topics related to TrustworthyAI.
Stay tuned for other valuable insights and groundbreaking research from our diverse community!
1. Safe Latent Diffusion: Mitigating Inappropriate Degeneration in Diffusion Models
Patrick Schramowski, Manuel Brack, Björn Deiseroth, and Kristian Kersting
2023, In Proceedings of the IEEE/CVF Conference on Computer Vision and Pattern Recognition (pp. 22522-22531)
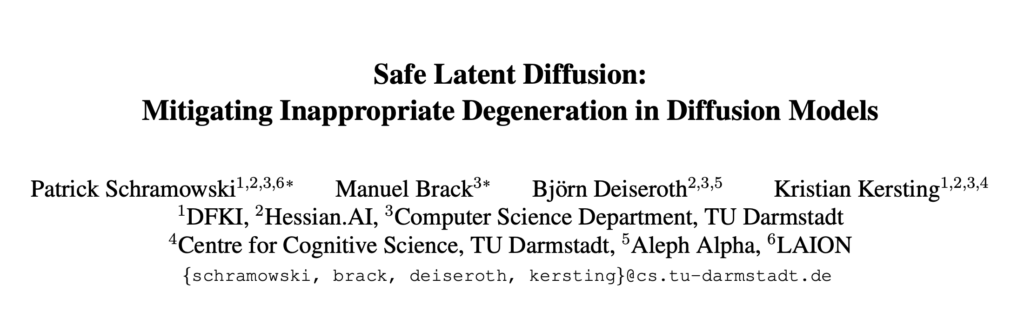
Abstract: Text-conditioned image generation models have recently achieved astonishing results in image quality and text alignment and are consequently employed in a fast-growing number of applications. Since they are highly data-driven, relying on billion-sized datasets randomly scraped from the internet, they also suffer, as we demonstrate, from degenerated and biased human behavior. In turn, they may even reinforce such biases. To help combat these undesired side effects, we present safe latent diffusion (SLD). Specifically, to measure the inappropriate degeneration due to unfiltered and imbalanced training sets, we establish a novel image generation test bed—inappropriate image prompts (I2P)—containing dedicated, real-world image-to-text prompts covering concepts such as nudity and violence. As our exhaustive empirical evaluation demonstrates, the introduced SLD removes and suppresses inappropriate image parts during the diffusion process, with no additional training required and no adverse effect on overall image quality or text alignment.
2. Human Action Recognition From Various Data Modalities: A Review
Zehua Sun, Qiuhong Ke, Hossein Rahmani, Mohammed Bennamoun, Gang Wang, and Jun Liu
https://ieeexplore.ieee.org/document/9795869?
2023, in IEEE Transactions on Pattern Analysis and Machine Intelligence, vol. 45, no. 3, pp. 3200-3225, 1 March 2023, doi: 10.1109/TPAMI.2022.3183112
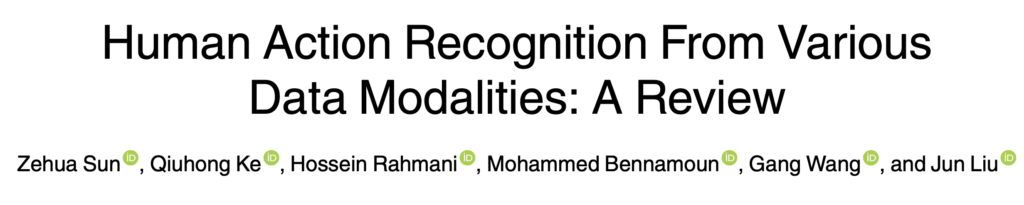
Abstract: Human Action Recognition (HAR) aims to understand human behavior and assign a label to each action. It has a wide range of applications, and therefore has been attracting increasing attention in the field of computer vision. Human actions can be represented using various data modalities, such as RGB, skeleton, depth, infrared, point cloud, event stream, audio, acceleration, radar, and WiFi signal, which encode different sources of useful yet distinct information and have various advantages depending on the application scenarios. Consequently, lots of existing works have attempted to investigate different types of approaches for HAR using various modalities. In this article, we present a comprehensive survey of recent progress in deep learning methods for HAR based on the type of input data modality. Specifically, we review the current mainstream deep learning methods for single data modalities and multiple data modalities, including the fusion-based and the co-learning-based frameworks. We also present comparative results on several benchmark datasets for HAR, together with insightful observations and inspiring future research directions.
3. Lifted inference for statistical statements in probabilistic answer set programming
Azzolini, Damiano and Riguzzi, Fabrizio
2023, In International Journal of Approximate Reasoning, 163, 109040.
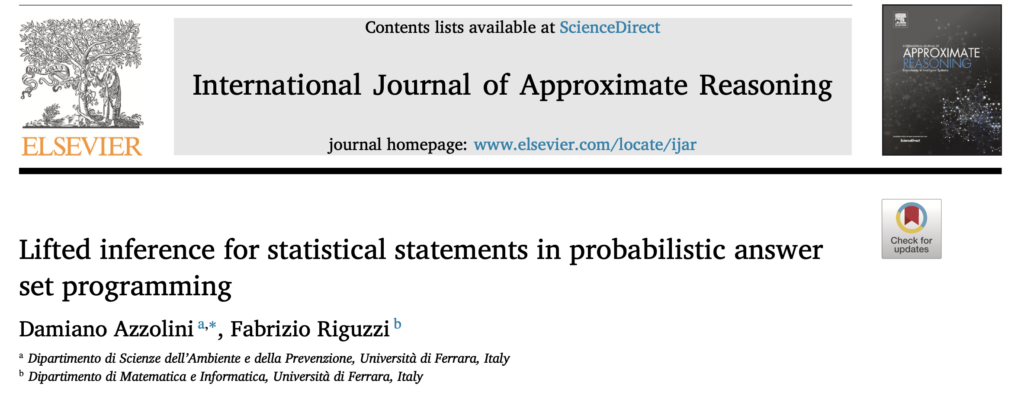
Abstract: In 1990, Halpern proposed the distinction between Type 1 and Type 2 statements: the former express statistical information about a domain of interest while the latter define a degree of belief. An example of Type 1 statement is “30% of the elements of a domain share the same property” while an example of Type 2 statement is “the element x has the property y with probability p”. Recently, Type 1 statements were given an interpretation in terms of probabilistic answer set programs under the credal semantics in the PASTA framework. The algorithm proposed for inference requires the enumeration of all the answer sets of a given program, and so it is impractical for domains of not trivial size. The field of lifted inference aims to identify programs where inference can be computed without grounding the program. In this paper, we identify some classes of PASTA programs for which we apply lifted inference and develop compact formulas to compute the probability bounds of a query without the need to generate all the possible answer sets.
4. Interactive Inference: A Multi-Agent Model of Cooperative Joint Actions
Domenico Maisto, Francesco Donnarumma, and Giovanni Pezzulo
https://arxiv.org/pdf/2210.13113.pdf
2023, in IEEE Transactions on Systems, Man, and Cybernetics: Systems.

Abstract:
Abstract: We advance a novel computational model of multi-agent, cooperative joint actions that is grounded in the cognitive framework of active inference. The model assumes that to solve a joint task, such as pressing together a red or blue button, two (or more) agents engage in a process of interactive inference. Each agent maintains probabilistic beliefs about the joint goal (e.g., Should we press the red or blue button?) and updates them by observing the other agent’s movements, while in turn selecting movements that make his own intentions legible and easy to infer by the other agent (i.e., sensorimotor communication). Over time, the interactive inference aligns both the beliefs and the behavioral strategies of the agents, hence ensuring the success of the joint action. We exemplify the functioning of the model in two simulations. The first simulation illustrates a “leaderless” joint action. It shows that when two agents lack a strong preference about their joint task goal, they jointly infer it by observing each other’s movements. In turn, this helps the interactive alignment of their beliefs and behavioral strategies. The second simulation illustrates a “leader–follower” joint action. It shows that when one agent (“leader”) knows the true joint goal, it uses sensorimotor communication to help the other agent (“follower”) infer it, even if doing this requires selecting a more costly individual plan. These simulations illustrate that interactive inference supports successful multi-agent joint actions and reproduces key cognitive and behavioral dynamics of “leaderless” and “leader–follower” joint actions observed in human–human experiments. In sum, interactive inference provides a cognitively inspired, formal framework to realize cooperative joint actions and consensus in MAS.
5. Token Boosting for Robust Self-Supervised Visual Transformer Pre-training
Tianjiao Li, Lin Geng Foo, Ping Hu, Xindi Shang, Hossein Rahmani, Zehuan Yuan, and Jun Liu
2023, in Proceedings of the IEEE/CVF Conference on Computer Vision and Pattern Recognition (pp. 24027-24038)
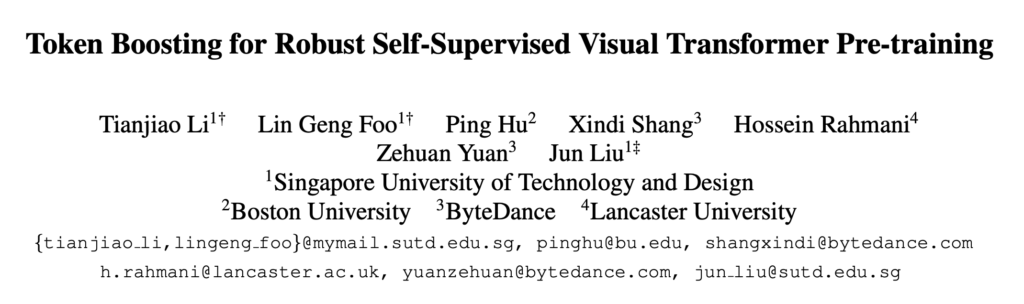
Abstract: Learning with large-scale unlabeled data has become a powerful tool for pre-training Visual Transformers (VTs). However, prior works tend to overlook that, in real-world scenarios, the input data may be corrupted and unreliable. Pre-training VTs on such corrupted data can be challenging, especially when we pre-train via the masked autoencoding approach, where both the inputs and masked “ground truth” targets can potentially be unreliable in this case. To address this limitation, we introduce the Token Boosting Module (TBM) as a plug-and-play component for VTs that effectively allows the VT to learn to extract clean and robust features during masked autoencoding pre-training. We provide theoretical analysis to show how TBM improves model pre-training with more robust and generalizable representations, thus benefiting downstream tasks. We conduct extensive experiments to analyze TBM’s effectiveness, and results on four corrupted datasets demonstrate that TBM consistently improves performance on downstream tasks.